AIIDE22: Neural Synthesis of Sound Effects Using Generative Models
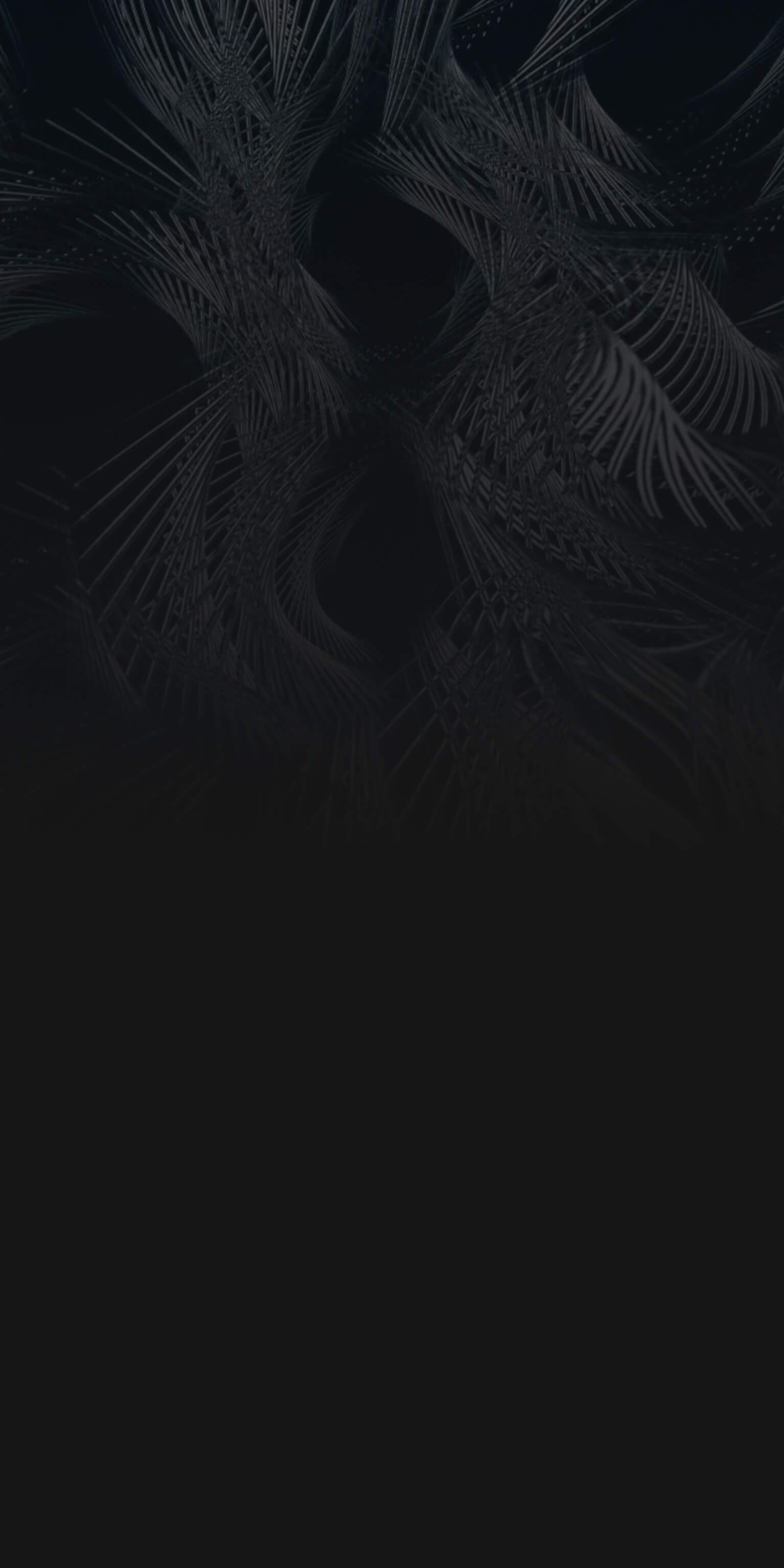
This research paper was presented at the 18th AAAI Conference on Artificial Intelligence and Interactive Digital Entertainment (AIIDE).
Authors: Sergi Andreu (KTH), Monica Villanueva Aylagas (SEED).
Neural Synthesis of Sound Effects Using Flow-Based Deep Generative Models
Download the full research paper (1 MB PDF).
Creating variations of sound effects for video games is a time-consuming task that grows with the size and complexity of the games themselves. The process usually comprises recording source material and mixing different layers of sound to create sound effects that are perceived as diverse during gameplay.
In this work, we present a method to generate controllable variations of sound effects that can be used in the creative process of sound designers. We adopt WaveFlow, a generative flow model that works directly on raw audio and has proven to perform well for speech synthesis. Using a lower-dimensional mel spectrogram as the conditioner allows both user controllability and a way for the network to generate more diversity. Additionally, it gives the model style transfer capabilities.
We evaluate several models in terms of the quality and variability of the generated sounds using both quantitative and subjective evaluations. The results suggest that there is a trade-off between quality and diversity. Nevertheless, our method achieves a quality level similar to that of the training set while generating perceivable variations according to a perceptual study that includes game audio experts.
