Multi-Critic Actor Learning: Teaching RL Policies to Act with Style
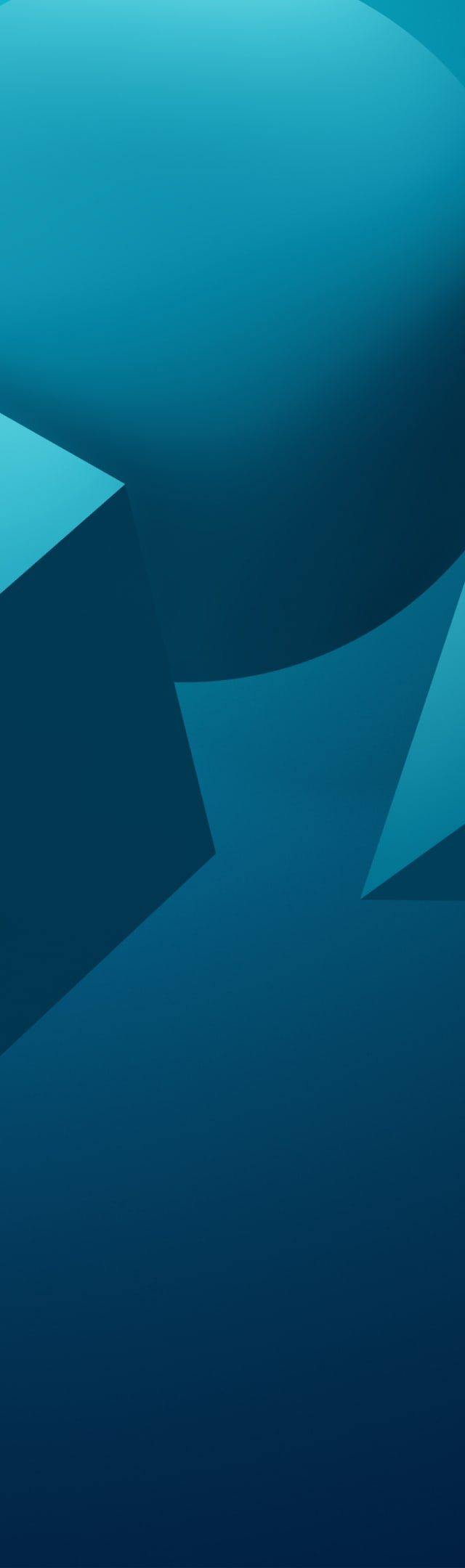
Authors: Siddharth Mysore, George Cheng, Yunqi Zhao, Kate Saenko, Meng Wu
Publication Date: 2022
Published in: International Conference on Learning Representations, 2022
Publication link: https://openreview.net/forum?id=rJvY_5OzoI
Abstract: Using a single value function (critic) shared over multiple tasks in Actor-Critic multi-task reinforcement learning (MTRL) can result in negative interference between tasks, which can compromise learning performance. Multi-Critic Actor Learning (MultiCriticAL) proposes instead maintaining separate critics for each task being trained while training a single multi-task actor. Explicitly distinguishing between tasks also eliminates the need for critics to learn to do so and mitigates interference between task-value estimates. MultiCriticAL is tested in the context of multi-style learning, a special case of MTRL where agents are trained to behave with different distinct behavior styles, and yields up to 56% performance gains over the single-critic baselines and even successfully learns behavior styles in cases where single-critic approaches may simply fail to learn. In a simulated real-world use case, MultiCriticAL enables learning policies that smoothly transition between multiple fighting styles on an experimental build of EA’s UFC game.
